ICIAM 2023
10th International Congress on Industrial and Applied Mathematics
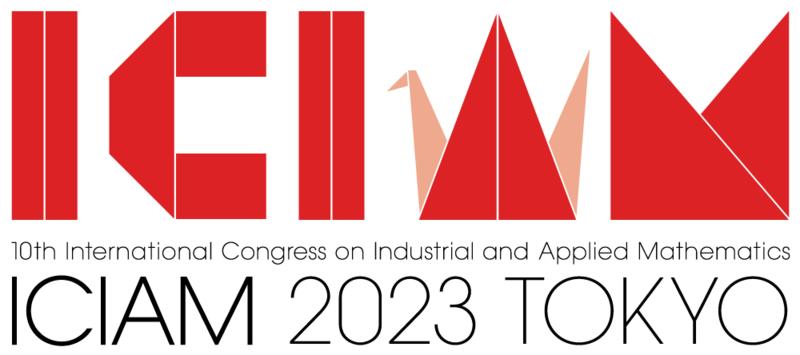
Sessions organised by members of the DataSıg team:
[00322] Methodological advancement in rough paths and data science
Rough path theory is an emerging mathematical technology that captures macroscopically interactions of highly oscillatory streamed data. Formally, it extends the domain of definition for the calculus of deterministic controlled differential equations, allowing them to be driven by complex signals, potentially rougher than Brownian motion. This area has built bidirectional connections with data science and machine learning, enabling the development of novel, mathematics-informed methods for efficiently analyzing time series data, e.g. PDE-based Signature kernel, path development layer with Lie group representation. This minisymposia series facilitates the discussion of new methodological innovations on this interface between rough paths and data science.
Speakers:
Christian Bayer | WIAS |
Thomas Cass | Imperial College London |
Ilya Chevyrev | University of Edinburgh |
Emilio Rossi Ferrucci | University of Oxford |
James Foster | University of Bath |
Satoshi Hayakawa | University of Oxford |
Chong Liu | ShanghaiTech University |
Qi Meng | Microsoft Research |
Hao Ni | University College London |
Harald Oberhauser | University of Oxford |
Josef Teichmann | ETH Zürich |
Danyu Yang | Chongqing University |
[00323] Integrating rough paths into domain applications
Streamed data are ubiquitous. In this context, a key challenge is to quantify our understanding and account for the interaction between channels. Rough path theory provides new insights for producing actionable inference for multimodal path-like data. The path signature is a mathematical object with desirable approximation properties and geometric interpretation which leads to more effective features and analysis. Further, the expected signature provides a powerful way to describe empirical measures on streams. Applications include award-winning machine learning methods in healthcare and finance, as well as commercial-quality Chinese handwriting software. We expose new challenges and work on applications in this area.
Organisers: Terry Lyons, Lingyi Yang
Speakers:
Paola Arrubarrena | Imperial College London |
Bruno Dupire | Bloomberg |
Elena Gal | University of Oxford |
Blanka Horvath | University of Oxford |
Mohamed Ibrahim | University of Leeds |
Florian Krach | ETH Zürich |
Darrick Lee | University of Oxford |
Maud Lemercier | University of Oxford |
Hang Lou | University College London |
Jason Rader | University of Oxford |
Benjamin Walker | University of Oxford |
Lingyi Yang | The Alan Turing Institute |
Other sessions with speakers from the DataSıg team:
[00059] Numerical solutions for differential equations: Probabilistic approaches and statistical perspectives
Many applications involve predicting the dynamics of a system by solving differential equations. Due to the increased demand for predictive power of these models, numerically solving a differential equation is now often combined with parameter estimation or uncertainty quantification. This paradigm shift drives the need for probabilistic approaches that are compatible with statistical inference, or that improve the robustness of inference to possibly inaccurate mathematical models. The talks in this minisymposium will present recent work that addresses these challenges for deterministic ODEs and PDEs, by using ideas from numerical analysis, probability theory, and Bayesian statistical inference.
Speakers include:
Yue Wu | University of Strathclyde |
[00201] Data-Driven Methods for Rough PDEs
Recently there has been an increased interest in applying data driven methods to learn partial differential equations (PDEs). For example, operator learning has been developed to learn maps between infinite-dimensional function spaces and has shown success in the context of smooth PDEs. However, these methods perform poorly in areas where PDEs are less well-behaved; for instance, when equations are parameterized by non-smooth functions or when the PDE involves stochasticity. This mini-symposium invites experts on novel methods for learning stochastic and ill-conditioned multiscale PDEs. Topics will include numerical methods for SPDEs, learning in multiscale settings, and advances in operator learning.
Speakers include:
Cristopher Salvi | Imperial College London |